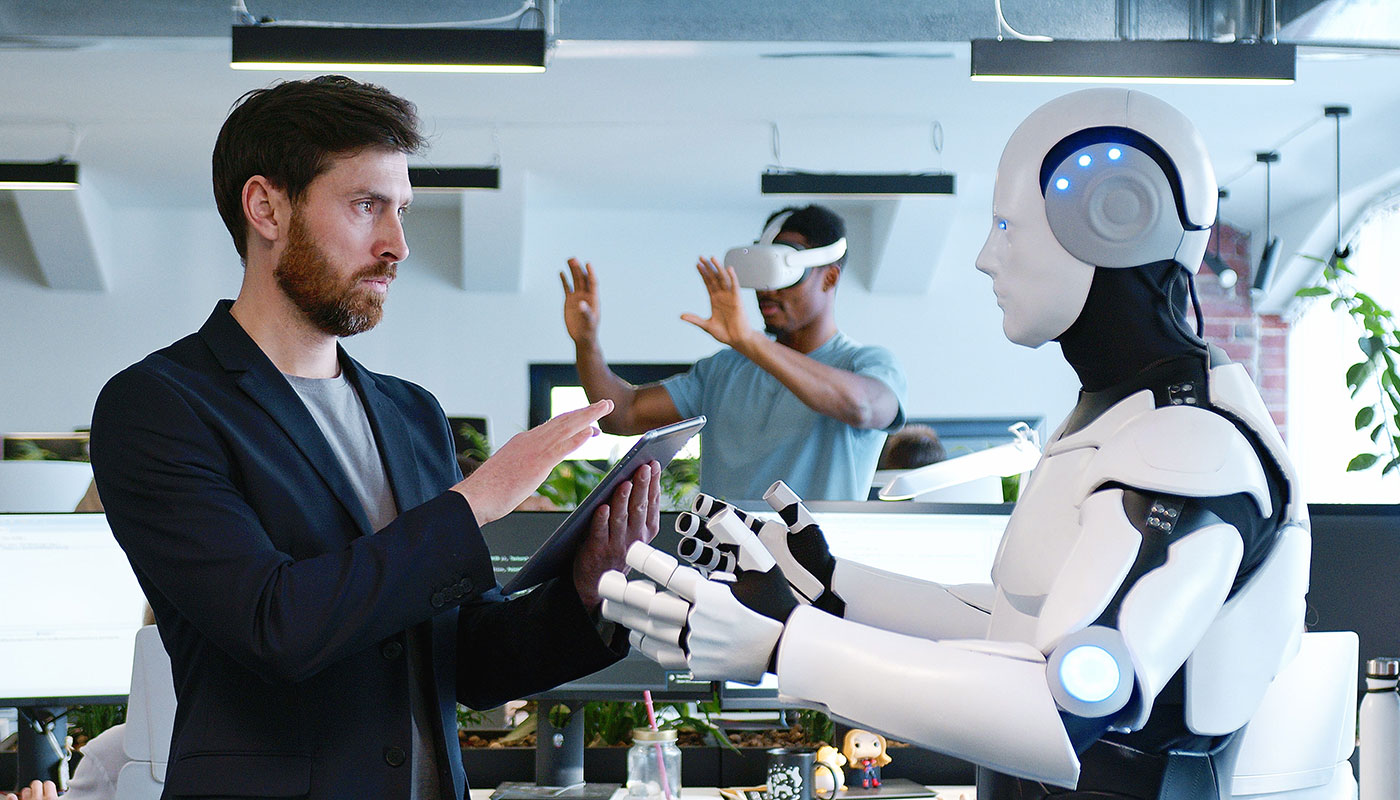
Resistive forces: examining counter-influences to AI uptake in IP practice
It is no secret that artificial intelligence (AI) is redefining industries, but its usage still stands at the crossroads regarding Intellectual Property (IP) and the patent landscape. On the one hand, large language models (LLMs) like ChatGPT, Bard and Alpaca intrigue us with the promise to automate much of the work of IP practice, from summarizing prior art to even writing parts of patent specifications. On the other, AI tools are still defined more by their limitations than their capabilities when it comes to matters of law.
Why LLMs cannot replace human legal experience
The disruptive potential of LLMs is undeniable: They can analyze documents and present information significantly faster than entire teams of people. But despite the time-saving opportunities afforded by such technology, it can act only as a supplement, not a substitute, for human intelligence and judgment.
Understanding nuances
Though LLMs can parse and generate text convincingly, they lack true comprehension. A patent's claims must be clear and concise while complying with the relevant patentability requirements. Only a trained patent specialist has the requisite skills to accomplish this reliably.
Legal responsibility
LLMs are neither natural nor legal persons and cannot be responsible for their activity. This bars them from owning property (including intangibles), entering contracts and assuming rights or obligations. By the same token, an AI cannot be held liable for any misfeasance or negligence, meaning this falls to a legally responsible party such as the owner, operator or developer. Essentially, jurisprudence safeguards the patent process as a fundamentally human activity — one governed by ethics, accountability and law.
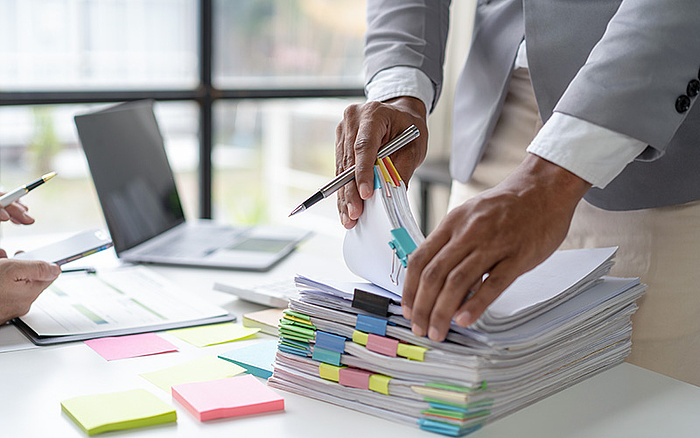
The legal system ultimately serves to regulate human behavior, whether by prohibition, mandate or punishment. Since AIs are only tools, it is the human operators, makers or owners of these technologies who must be bound by the law to ensure safe, fair and accountable use.
Decision-making
Humans use reason, conscience, precedent and intuition in coming to their conclusions, to say nothing of emotion. These are complex faculties that LLMs cannot replicate, least of all in determining the scope of patent claims or concluding where the risk of infringement exists.
Semantic pitfalls in patent drafting
As mentioned, LLMs can review texts and replicate language in an almost invisibly naturalistic way. The sentences they piece together are grammatically correct and contextually appropriate, but when discerning the subtleties of specific legal terms, complex interactions or complex technologies, they falter. This is because semantic analysis — the ability to analyze words and phrases — is fundamentally different from linguistic comprehension, the ability to grasp the actual meaning of those words. The generator has no apprehension of the words used, identifying only that they fit within observed patterns.
When applied to patent drafting, the results of this limitation are magnified. LLMs may produce patent claims that are superficially sound but may harbor issues such as overly broad language, misrepresentation or illogical technical features. The inability of AI to understand the implications and gradations of legal language means that, if AI is to be used at all, a human expert must still review, edit or even completely rewrite AI-generated content.
The economic balance of the IP sector
The IP sector operates like an enclosed economy, with its own ecosystem of actors and stakeholders: Inventors who create new innovations, the legal experts who navigate patent registration, prosecution and enforcement and the governmental or organizational bodies that grant patents and arbitrate disputes. This structure has been finely tuned over decades, and its stability is crucial for promoting innovation.
As AI technologies increasingly penetrate each segment of this setup, the possible repercussions are both monetary and operational. AI-driven processes could disproportionately benefit certain stakeholders at the expense of others, thereby upsetting this carefully calibrated, if imperfect, equilibrium.
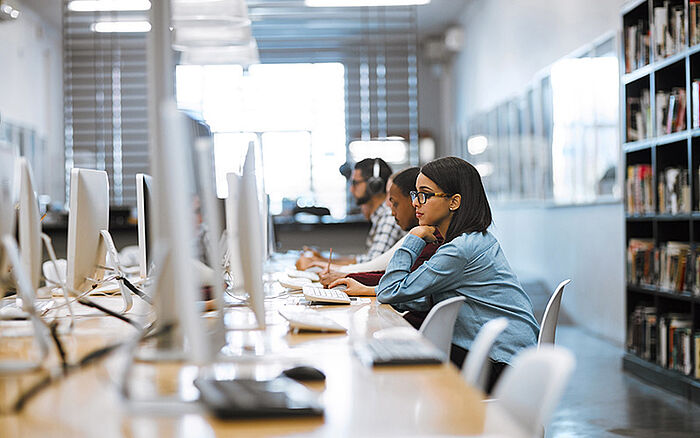
Disruption is not always good for innovation. An over-reliance on AI within IP procedures risks damaging quality control. Alternatively, smaller, independent developers could be priced out of the patent system if offices struggle to cope with an upsurge in non-human-generated inventions.
- The inventive process: Excessive reliance on AI models to generate marginally improved inventions could result in an overwhelming surge in patent filings. Inundated, patent offices would experience a severe bottleneck that could delay registrations and disrupt the entire patent-granting pipeline. To offset this, offices would likely be obliged to take on more staff and increase their fees.
- The drafting and filing process: If AI technologies automated all tasks traditionally carried out by legal experts, the average quality of patent applications would drop, and volumes increase. Without a suitable screening stage before patent filing, IP offices would again be overburdened, creating another negative feedback loop of rising costs.
- The examination process: Due to their linguistic shortcomings, the use of AI in substantive examination procedures could lead to lower-quality patent grants. Subsequent infringement and revocation actions could clog the courts, siphoning off a reduced pool of legal expertise and driving up expenses for both patentees and defendants.
Any combination of the above results in a very "noisy" IP scene that potentially multiplies the complexity of litigation and inhibits the very knowledge-sharing that is the raison d'être of patents. However, the solution is not to dismiss AI out of hand and remain stagnant; using AI in certain procedures and processes could lead to cost and time savings. Instead, the IP sector needs to embrace AI tools in such a way that they remain just that: tools.
If utilized judiciously and with human oversight, AI has the capacity to provide valuable support to legal experts in their daily activities. With appropriate use, these technologies could lead to a more efficient, accessible and affordable patenting system in the not-too-distant future.
A version of this article was first published on IP Service World.
Filed in
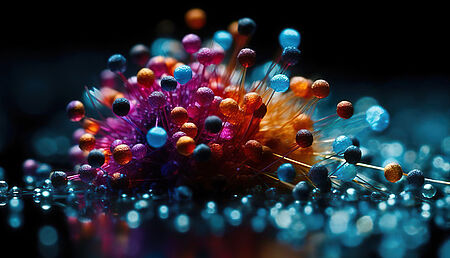
Take a closer look at some of the top pharmaceutical trends and how IP is driving and being driven by these changes.